Part One
The insurance industry has a conservative way of doing things. Unlike its city partner the finance industry, insurance has been slow to embrace technology.
Banking clients used to suffer poor customer experiences and the stock market was just men in a room trading shares, but now banks like Monzo pride themselves on customer service and the LSE has become a digital exchange. Hyperfast, computer-powered trading outfits now account for about half of all US equity trading, according to Credit Suisse - evidence that the sector is far outpacing the sophistication of the insurance industry.
There are signs that this is changing, however; customers are demanding more personalised and meaningful services and are using their smartphones to purchase insurance more than ever, meaning insurers really have to catch up if they want to stay relevant.
Add to this the increasing availability of consumer and commercial data and the tech industry’s ever-growing capabilities and it’s clear that those insurance companies who stick to a traditional system will soon fall behind in the market.
However, change means disruption - which in turn means costs.
So modern insurers are facing a dilemma: how can technology be used to improve an insurance business without completely overhauling its existing structures?
Modernising product pricing
Take pricing. Traditional insurance pricing models are largely simplistic - a pricing matrix system, for example, registers the price of a consumer product and alters the premium accordingly from a couple of variables, categorising the risk in a simple matrix.
These models can sometimes lead to uncontrollably high exposure and loss ratios, as there is no guarantee that the simple pricing formula is indeed linked to the actual risk (i.e. the level of claim).
The lack of additional data input, as well as a strict set of constraints, means that insurers miss out on a level of accuracy that could potentially be achieved with technological assistance. In order to be more accurate, the pricing should theoretically take into account more variables. Indeed, the number of internal and third party data sources available is increasing all the time.
It seems reasonable to suggest that Machine Learning, with its ability to learn virtually any patterns with great flexibility from a large amount of risk factors, could improve the way these pricing models work and solve a lot of headaches for insurers.
So if it’s that simple, why hasn’t the whole industry already made the switch to AI pricing?
The traditional approach
Let’s look at the banding approach to pricing, where premiums are assigned from only one or two simple variables. In this model, the price of a product alone could determine its premium, so the more something costs the more the consumer pays (within premium brackets, or ‘bands’).
The problem with this as a model is that the premium does not take into account other variables that may relate to the risk, such as location or previous claims. This could mean a customer with a history of claims buys insurance for the same price as someone without.
A rigid pricing structure can also lead to higher loss ratios. If a pricing band covers a large range, the majority of customers will end up buying the same price cover whether they’re at the top of the bracket or the bottom; those with a higher risk can end up in this lower pricing level because of simplistic variables, and loss ratios are substantially higher than they need to be.
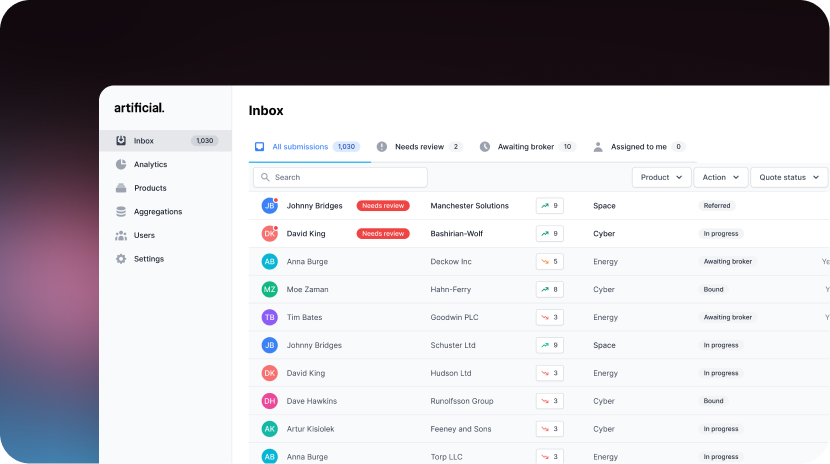
The drawbacks of blunt Machine Learning
Theoretically, adding more variables would create a more accurate assessment of a risk, leading to a better pricing model for consumers and reduced loss ratios for insurers: a win-win, right?
Not so much.
For an insurer who already has a commercialised product on the market with an established pricing structure, Machine Learning can just create more problems that it solves.
Yes, insurers may love the sound of complex algorithms and fancy processes, but the reality is less straightforward.
Switching to Machine Learning blindly can create a sort of ‘black box pricing’ model. If an ML pricing engine is fed with vast amounts of data and variables, it becomes instantly less transparent to the insurer and less explainable to the customer. A price generated by 20 different factors becomes very difficult to explain to a consumer who wants to know why he’s suddenly paying more than his neighbour for the same product.
Most Machine Learning pricing engines also ignore the structures an insurer already has in place. If a pricing model has been established for a product that’s already on the market, it’s hugely inconvenient - and costly - to throw it out and start again.
As well as this, typical ML pricing engines only evaluate and illustrate the benefits of these models by usual ML metrics, which are not friendly to an insurer and may not be very convincing to actuaries. Without evaluating and optimising the pricing engine in a business and actuarial context, the engine could perform well in model training but have a biased prediction when it’s actually implemented.
What now?
In order for an insurer to adopt Machine Learning, it needs to really be worth it. Algorithms and processes have to be appropriately managed and adjusted, which can be complex, and the technology used needs to show real results in real scenarios.
Insurers need access to the right data and the best tools to implement Machine Learning, as simplicity and a lack of nuance means that all the potential benefits are lost.
Next week we will explain how the team at Artificial are using Machine Learning and AI to improve pricing models for insurers within their own established structures.
For more information, get in touch.
References
The quickening evolution of trading — in charts: Financial Times https://www.ft.com/content/77827a4c-1dfc-11e7-a454-ab04428977f9