What is algorithmic underwriting?
Algorithmic underwriting essentially means making automated decisions based on the data at the user's disposal.
It gives insurers the power to automate the process of taking on risks and allows them to customise their own bespoke rule set and risk appetite, taking on only those policies which match their specific criteria without having to spend hours scanning policy information documents first.
At Artificial, we've developed an algorithm that underwriting teams can use to scale the process of choosing high-quality risks (consuming large amounts of data and an increased number of submissions) without adding people and resources - freeing up their time to spend on value added tasks.
Decisions can be rejected or accepted automatically, or referred to an underwriter for further investigation (human-in-the-loop).
The key to applying algorithms to this kind of complex underwriting is manifesting digital risk data in a structured way. These algorithms can support decision making at any given level of detail and complexity, as long as there is enough good quality data present.
Third-party data providers can be used to augment and validate risk data automatically and defined rules can be applied automatically using a digitised risk submission.
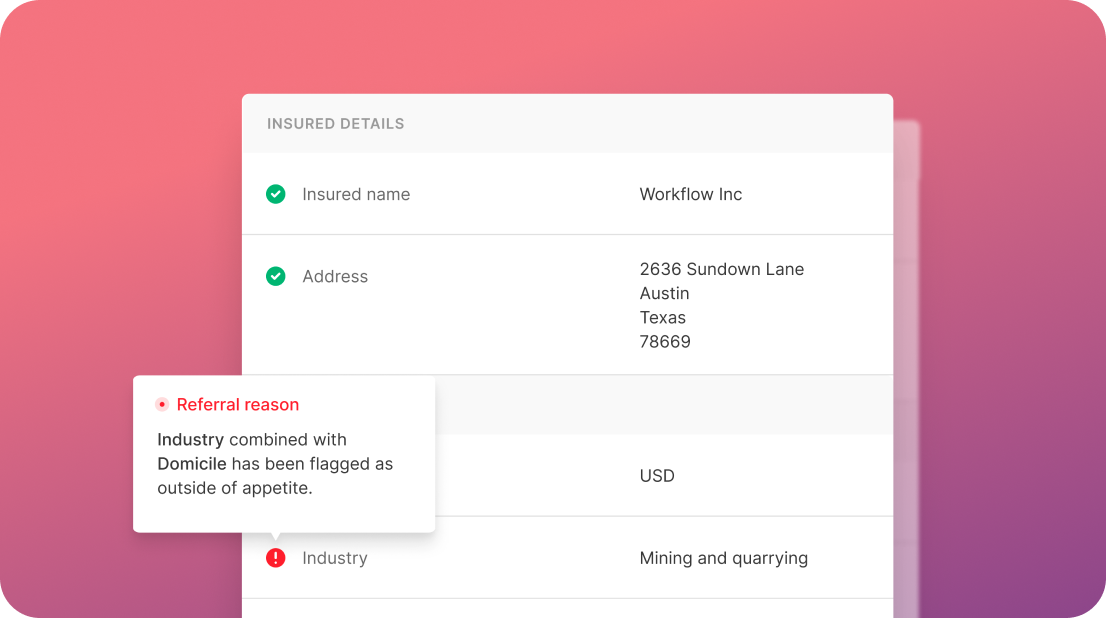
How algorithmic underwriting helps carriers
Algorithmic underwriting can work for both follow and lead business, but both ultimately help the carrier in similar ways.
Smart Follow
Smart Follow allows follow underwriters to put their trust in lead underwriters. They can choose to automatically accept risks written by certain underwriters because they know that they share a similar risk profile based on several consideration factors.
The decision of whether to follow business is based on information contained within the underwriting slip and related attachments (schedules of values, loss records and so on).
The technology allows underwriters to choose a bespoke set of rules to follow, ensuring that they only take on syndicated policies which match specific criteria.
Underwriters can therefore manage both time and risk simultaneously, making savings along the way and passing those savings onto customers to increase value across the service as a whole.
Smart Lead
In Smart Lead underwriting, the data helps an underwriter decide whether or not to write a lead line. Usually the lead puts significantly more work into understanding a risk because they are in the position to set terms and rates, so with algorithmic underwriting decisions can be informed at a very granular level in a fraction of the time.
Lead underwriters can use the technology to add even more value, by digitising risk data and sharing with their follow market. This would allow them to be both an enabler of Smart Follow and a leader who helps build a more efficient marketplace.
Although the interest in Smart Lead is undoubtedly less than Smart Follow, there is scope for its increased use in the future as the technology is developed (and accepted by the market).
The technology used to enable algorithmic underwriting can be restricted by the amount and quality of structured data available, but as the trend grows towards digital sharing–for example in Blueprint 2–more complex algorithms can be put in place. The more information that underwriters receive, the more data can be analysed.
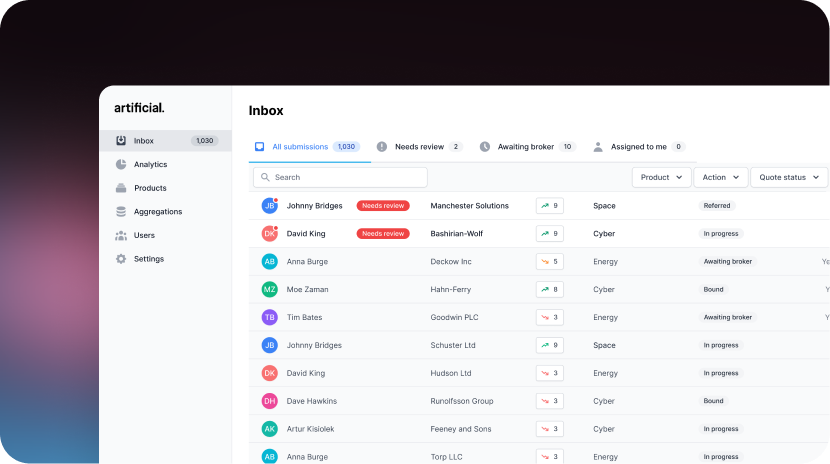
The benefits of algorithmic underwriting
There are several benefits to be gained from algorithmic technology in both immediate and future terms:
1. Scalability
There is an advantage to putting the infrastructure in place and using it for simple decision making in the short-term in order to make efficiency gains.
For example, an underwriter could decide not terms but just determine if the risk type, location etc are acceptable. This should be done with the knowledge that, as more data becomes available, the tool can be rapidly scaled.
Once one product is codified, it becomes easier to scale other products without needing to add more staffing resources.
2. Tailored technology
Underwriters can tailor algorithmic technology to work for them, no matter what their business model looks like.
Not only does this mean that decisions about insurance syndication can be made more accurate, but it also frees up underwriters to spend less time on basic administration and more time on judgement-heavy tasks.
Risks that are either clearly within or definitively out of appetite receive an automatic response, so underwriters can focus their time on reviewing the remaining opportunities for which it’s not possible to make an immediate decision.
3. Future-proof
Digitising and storing all initial submission information to create a digital library allows for more detailed interrogation in the future.
Current technology enables the use of a lot of the shared information, but as models improve more insight can be gained from this information. This also allows for retrospective analysis, e.g. including a pandemic clause.
4. Customer satisfaction
Finally, the benefits of this approach are also passed down to brokers, who experience faster turnaround times and become happier, more productive clients whose ways of working are uninterrupted.
Brokers can pass this onto the insured who in turn experiences an even better service.
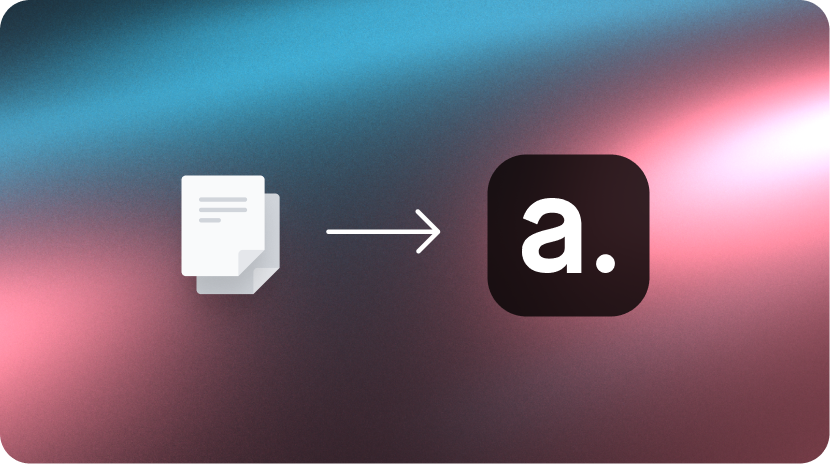
Why are complex products difficult to automate?
While other less data-intensive sectors of underwriting have already been reaping the benefits of automation for some time (you can read more about this here) many insurance companies and syndicates are yet to embrace algorithmic underwriting and machine learning.
When it comes to complex risk, policy information currently doesn't usually exist in a standard digital structured format. Instead, most of the details of policy information are contained within PDFs and other hard-to-digitise formats.
If data like this isn't accessible to a computer, decisions can't be automated because there is insufficient information available for the computer to apply the relevant rules and make smart decisions.
It's clear that in order to successfully automate follow business for open market commercial insurance, it's first necessary to make this policy information accessible to the rules engine, as well as to ensure that underwriters remain in control of the decisions it makes.
How our algorithmic technology works
We use machine learning technology and proprietary models to digitise and structure insurance policy documents. In other words, non-structured data can be quickly and accurately captured and organised into a standardised format, making policy information available for efficient analysis and decision-making.
By combining this technology with our knowledge of digital standards and insurance expertise, we can enable underwriters to configure their appetite based on relevant factors, making it as simple or as complex as the underwriter requires.
Our machine learning technology can be used to standardise policy information and incoming claims data, enabling underwriters to scale whilst freeing up their time for high value tasks. This could be via email or e-placement submissions, both of which enable the broker to continue to operate as normal with the benefit of behind-the-scenes technology.
Get in touch with us
Algorithmic underwriting is just one example of the many ways in which automation and machine learning can be used by insurers to improve working practices and add value to their work.
We've written extensively in the past about how machine learning can be harnessed in underwriting, including to improve companies' pricing systems and the benefits of human-computer collaboration.
If you're an underwriter interested in taking on more insurance syndications and you think our algorithmic underwriting tool might be for you, get in touch with us. We'll be happy to discuss the capabilities of our technology with you in more detail, and help you to see the ways in which algorithmic underwriting could help you to flourish in the digital age.