In Part One we looked at the pitfalls of using a one-size-fits-all approach to Machine Learning. In Part Two we focus on how the team at Artificial are using Machine Learning and AI to improve pricing models for insurers within their own established structures and frameworks.
We learned in Part One that, if used with reckless abandon, Machine Learning can create more problems than it solves. Black-box pricing, unclear results and complicated metrics all explain the current lack of AI adoption in the insurance sector, particularly compared with other financial industries.
Why then, you may ask, should an insurer even bother with the switch to AI pricing?
The answer is that when implemented carefully for an insurer’s specific needs, AI can be used in a multitude of ways to aid the insurance lifecycle.
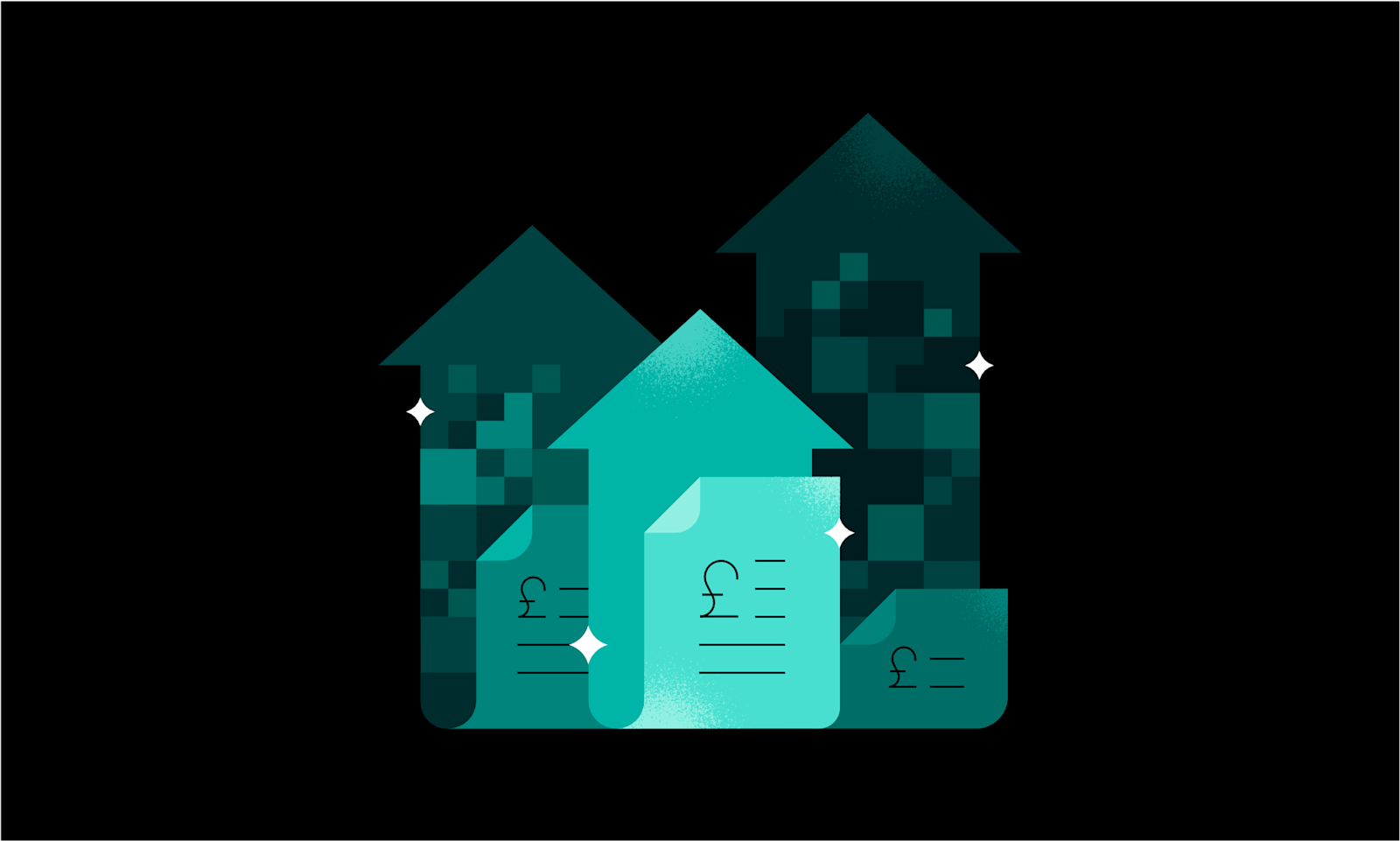
The benefits of (clever) AI pricing
One of the benefits of a comprehensive Machine Learning pricing engine is that it can improve the accuracy of pricing for customers, giving them fairer premiums than with non ML-based models.
Take motor insurance: with a traditional pricing model, a younger driver may find themselves faced with higher premiums simply due to their age when in fact they drive safely and are a low risk. If an insurer using an ML-based pricing model can take into account other variables that indicate this lower risk, the young driver has a lower premium and is more likely to choose that insurer.
This leads to increased customer satisfaction, a greater sense of loyalty to the company and, ultimately, higher retention rates (a notoriously sore spot in the insurance sector).
A tailored ML algorithm also works wonders for improving risk sensitivity. Where a simple matrix-based system only predicts risks based on a couple of variables, an ML pricing engine can find patterns in claim data and link it to a customer or policy characteristics. New customers are then charged appropriately according to these improved predictions.
This enhanced risk sensitivity translates to better profitability for the insurer and a more controlled and lower loss ratio. ML-based pricing, when implemented properly, can also allow insurers to adjust their prices dynamically to meet certain financial targets and adapt to market competition.
However, as we saw in Part One, it’s not that simple to make the switch to AI pricing. Disruption means time and money, and most companies don’t want the chaos of upgrading their whole system and processes.
At Artificial, we develop systems to implement Machine Learning pricing for both new and existing consumer products without the chaos of a restructure - showing that, in the right hands, AI pricing can be hugely beneficial to the insurance industry.
The Artificial solution: data is everything
Before a new pricing model can be set up, an insurer needs enough claim and policy data to feed into the ML engine. Data is the fuel for any ML model, and there’s no point in implementing a smart new system if there’s nothing to go into it. And the quality of data - its completeness, its level of detail - is as important as its volume.
But the process of capturing this data and converting it into a format that is consumable by a pricing model can be time-consuming. Information can come from anywhere, from traditional claims and policy management systems to more fragmented sources such as photos, surveys or even paper-based sources.
To streamline this process for insurers, Artificial systems capture data digitally from the necessary sources before converting and feeding it into our pricing engine. Furthermore, this data can be analysed to research things like causes of claims and unexplored risk factors.
At Artificial we recently performed an analysis based on telematics data showing that, in the case of motor insurance, one of the highest causes of claims was due to a significant change in driver altitude.
So for example, if a driver went skiing for the weekend and drove up and down mountains, they were much more likely to claim than someone with a lower change in altitude. When you combine this data with other sources such as weather data, you begin to paint a much clearer picture of the underlying risk. It’s hard to see how this sort of analysis could be done by humans without painstaking research and a lot of time and effort.
Existing structures
How, then, do we use this information in a real-life insurance context?
It’s safe to say most existing motor insurance pricing structures don’t take into account the change in altitude of a driver, or many other significant data features that may be better indicators of underlying risk.
To reap the benefits of analysis such as this – with minimal disruption – Artificial is able to adapt its pricing engine to an insurer’s current pricing structure.
For example, if the premiums for an insurer's existing consumer product are set into five pricing bands (see Fig. 1), our ML pricing engine can assign future premiums within the same universe of those five bands, therefore keeping the insurer’s existing systems and pricing structures in place.
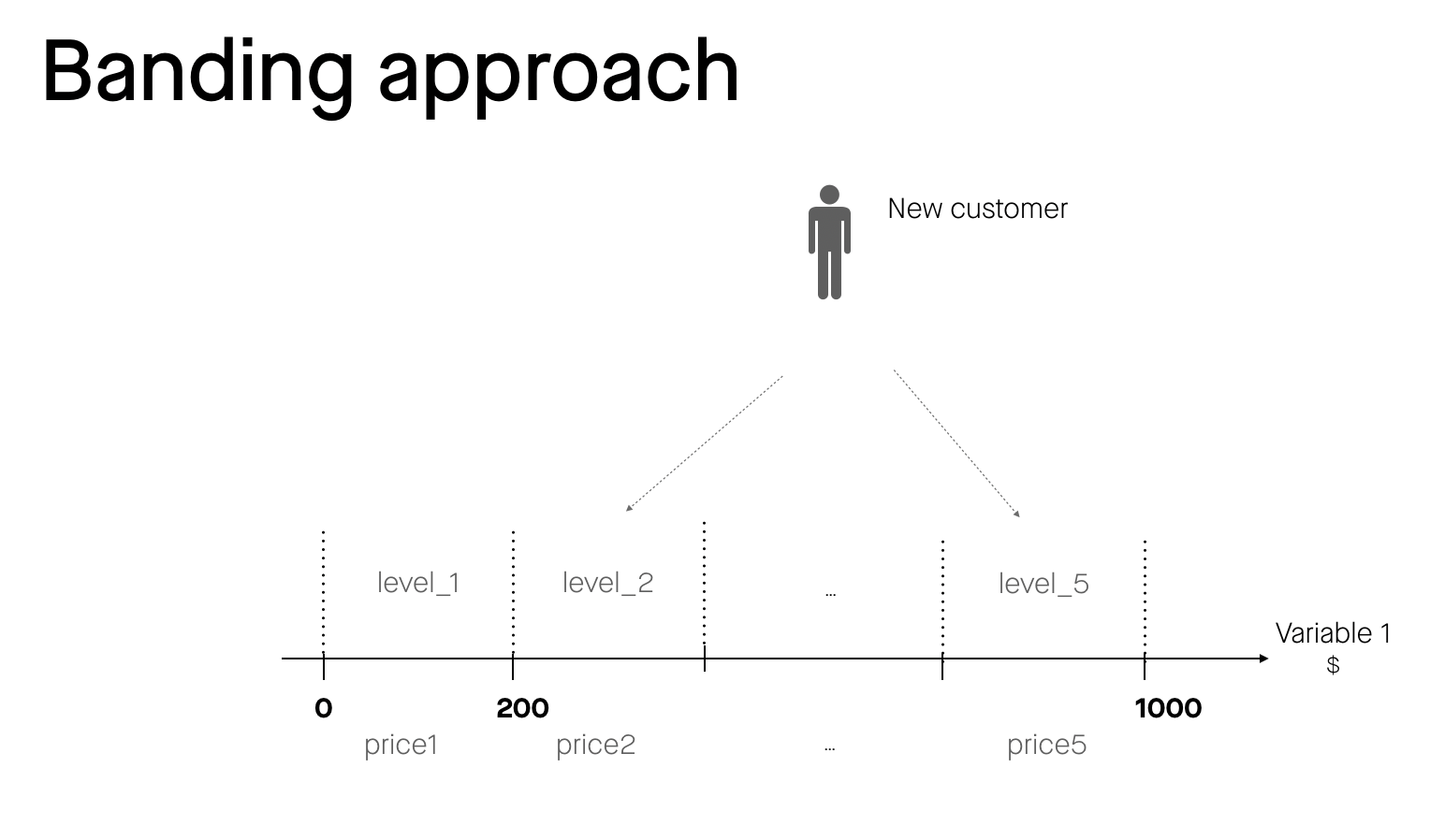
Fig. 1
In this case, only the allocation of customers into the different pricing bands is changed, not the prices themselves. The ML engine is used to do the allocation, making it risk sensitive and ensuring the optimal price band is chosen for all customers considering their predicted risk. This ensures minimal disruption to current processes but at the same time is a highly tractable system.
Additionally, adjustable parameters can be set within these structures to constrain the amount the ML allocation can deviate from original prices. Prices for each band can be moved up or down by a certain percentage to meet loss ratio targets, and limits can be imposed on the allocation of new price bands (so, returning customers could be allocated in only the next/previous price band than before, not jump from the first band to the last).
This allows a smooth transition to AI pricing for the insurer but with the necessary levers for tweaking to increase profitability or react to market competition over time.
More transparency
As discussed in Part One, one of the presumed benefits of AI-based pricing is that an ML engine can take in many more variables than a simplistic, Excel-based model, leading to a more accurate assessment of risk. However, the addition of all of these extra variables instantly means any premium generated is less transparent for the actuary and ultimately the customer - the ‘black-box pricing’ model.
At Artificial, we put transparency at the forefront of the user experience. In clarifying the variables behind each assigned premium, customers have a clear explanation of why their products are priced at a certain amount.
Furthermore, insurers are able to further understand the pricing engine and assign appropriate future prices based on key risk variables.
Improved profitability
When used in the correct way, one of Machine Learning’s best achievements in insurance could be the improvement of loss ratios.
We’ve seen how more variables can better the assessment of risk, but at Artificial this is taken a step further. If we go back to the banding model, risk sensitive allocation means that ‘riskier’ customers are assigned to a higher price band and conversely, lower-risk customers to a cheaper band.
Over time and with the input of more data, the ML engine learns patterns and improves risk allocation, bringing with it more ‘good’ risks and fewer high risks in the insurer’s portfolio. All of this leads to a reduced loss ratio - the struggle for which is surely one of insurance’s biggest headaches.
A real-world example of how an Artificial pricing engine helped improve an existing consumer product loss ratio for an insurer is given below (Fig. 2).
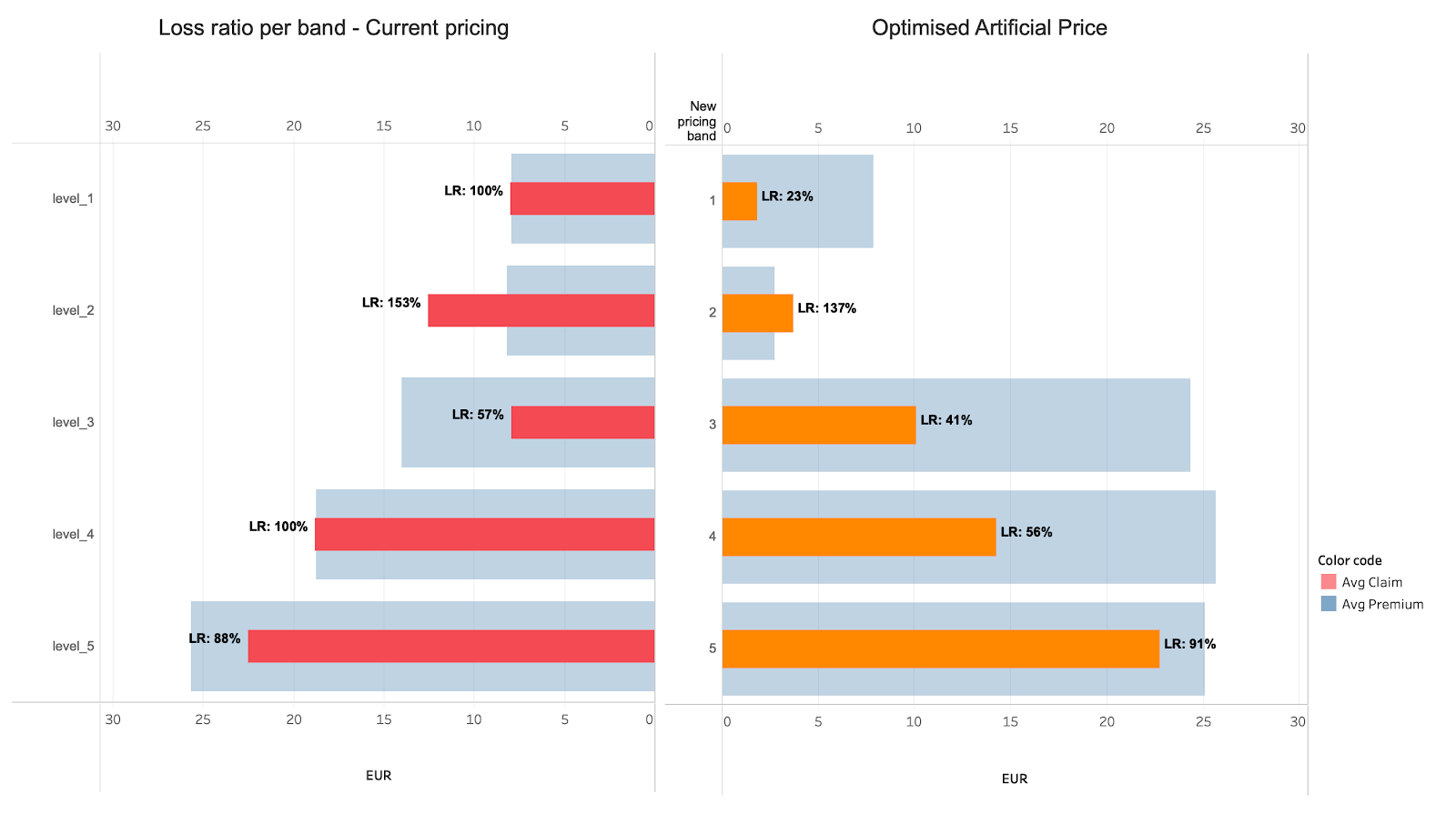
Fig. 2
In this case, we plugged our ML engine into the current pricing structure of the insurer. We did not change the number of price bands, nor the price for each band, ensuring minimal disruption to current processes. By just using our engine to reallocate customers into a more optimal band, we achieved an ~18% improvement (decrease) in loss ratio overall for the insurer.
Looking ahead
What does all of this mean for the future? It’s not inconceivable that customer behaviour will be influenced by changes in pricing. Parametric insurance (or ‘on demand’ insurance) will be vital to this; in the driver altitude example, environmental factors may deter customers from driving in a particular location or if the weather is bad because their on-demand premium could rise as the risk of claim becomes higher.
Even so, there is no way of knowing how customers will perceive this new approach. Changing pricing models in the industry is one thing, but assessing the way customers react to that particular change is another.
How to determine the impact on profitability and the respective price elasticities of a product’s offering, particularly across an insurer’s entire portfolio, is perhaps the most important question for insurers (at Artificial, we’re developing a model to assess and predict this – but that’s an article for another day).
Conclusion
So with time rapidly edging towards 2020 it’s certainly expected that more and more insurers will look to switch to AI pricing in the coming years.
But we know that not all insurers are the same. Some may be starting their ML pricing journey from the very beginning - with entirely paper-based processes and few ideas on how to progress - and some may already have basic digital structures in place.
Artificial aim to use Machine Learning and AI cleverly, making it fit into an insurer’s current way of doing things rather than causing chaos and changing all of the processes around it.
We want to provide better user-end tools from a grass roots level that improve an insurer’s day to day operation, capturing data digitally and enabling them to leverage ML-based pricing, no matter what structures they currently have in place.
Many companies will offer some sort of Machine Learning pricing tools, but few will actually make it helpful and practical for insurers – instead implementing poorly thought out systems that hinder rather than help.
As more and more insurers make this transition, it is those companies who fully understand the idiosyncrasies of AI pricing that will surely profit the most.
For more information or to see how we could help you, get in touch.